A Review of the the Use of California Department of Developmental Service’s Autism Data
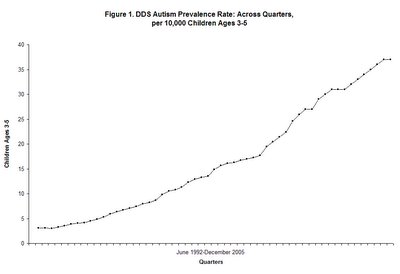
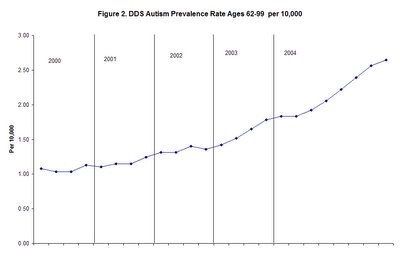
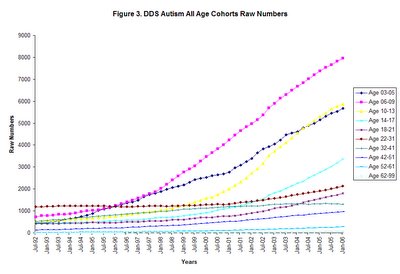
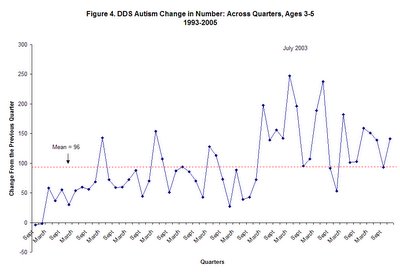
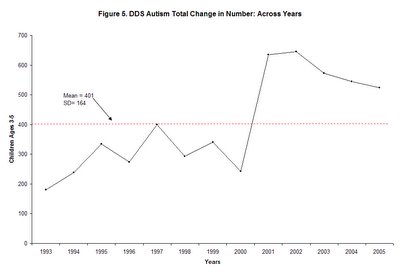
Abstract
The California Department of Developmental Services (DDS) quarterly reports have been used by some to calculate incidence of autism. The California DDS has explicitly stated that their data should never be used to calculate the incidence or prevalence for autism. In the current post the implications for this are discussed. This post will also include a more thorough analysis and discussion of the DDS autism data than has been offered in previous reviews on this site. The results indicate that the DDS and descriptive epidemiology appear quite different. The conclusion from this analysis is that the California DDS data which the DDS states should never be used for descriptive epidemiology purposes and which does not resemble existing epidemiology, should not be used as epidemiology.
Introduction
The California DDS system is a voluntary system that provides services to persons with disabilities within the State of California. It does not provide services when such are not requested, nor does it accumulate data on such persons (Department of Developmental Services, 2005).
For those who enter the system, the DDS does compile certain data. This includes things like age, Developmental service category, and certain co-morbidities (DDS, 2005). These data are useful when making reports to law makers or consumer advocates who wish to see for whom, resources are being allocated.
Among other categories, the DDS serves autistic children and adults, who are at least 3 years old. This system’s collection of data over the years, make it appealing to those who wish to calculate prevalence or incidence or autism. This suddenly becomes an issue when we consider the claims of a current autism epidemic (MSNBC, Autism: The Hidden Epidemic, March 3, 2005).
Within the peer reviewed literature the DDS data have also been used to argue for an autism epidemic (Balxill, Baskin, & Spitzer 2003). These data have also been used to help support an argument for a proposed decrease in new intakes of children receiving services for autism (Rollens, 2006). The data have likewise been used to support the idea of diagnostic substitution as the source of the apparent increase of autism (Croen, Grether, Hoogstrate, & Selvin, 2002).
The changes in the DDS data have been argued as proof of an autism epidemic now in recession due to the removal of thimerosal (Rollens, 2006). This is unfortunate as epidemiology can not establish causation (Friis & Sellers; Last, 1995; Taubes, 1995; and Winkelstein, 1995). It seems prudent then to show some restraint when discussing what epidemiological data indicate.
The fact that the DDS has argued against this use of their data for epidemiological purposes, has been stated as a valid reason to discard such analyses http://interverbal.blogspot.com/2006/01/review-of-california-department-of.html. As these data continue to be used as formal proof, this post will include an analysis of the failure to account for threats to internal validity and the presence of problems with external validity. Problems with random and systematic error will also be discussed. The presence of these uncontrolled threats, as well as the differences from existing descriptive epidemiology, offer a strong agreement with the statements by the DDS, that these data should not be used to calculate incidence or prevalence.
Methods
The DDS autism data were taken from the quarterly reports (DDS, 2006) and from personal communications with the DDS. Additional data were taken US Census Bureau (USCB) projections and the 2000 census (USCB, 2006). Data were calculated to form a prevalence rate per 10,000.
The DDS data served as the denominator and census data as the numerator. (10,000) was then divided by the outcome to give the rate per (10,000). As there was numerous data entry and calculations used this process an additional student checked for accuracy of data entry and calculations on all relevant points. There were no disagreements, inter-observer agreement equaled (100%).
The analysis was conducted by visual inspection of the data path in line graph format as generated from MS Excel. The data path was assessed for relevant patterns such a cyclical trend, epidemic threshold, and case clustering (Friis & Sellers, 2004). Mean and standard deviation data was calculated as an additional descriptive tool for the net, quarterly report data changes.
Analysis
Figure 1. shows the prevalence rate of 3-5 year olds as calculated from the DDS data and the USCB. The increase is steady with the last two data points having the same rate, even though the raw numbers continue to show an increase between those points. This is not indicative of slowing of the increase as other points in the data path are also the same or very nearly the same.
The 3-5 cohort is the youngest group via the DDS that we have adequate data on. To serve as a counter balance I have presented Figure 2. which follows the oldest cohort of ages 62-99. An upward trend is also noted for this group, but at a much lower level. The cause of this in unknown, but this could be accounted for by diagnostic substitution for persons who were already in the DDS.
Diagnostic substitution from mental retardation to autism may not be wholly sufficient to explain the change (Blaxill et al., 2003). This author is also analyzing those data, and the analysis seems to support Blaxill et al. (2003) in that not all the change between categories can be accounted for by shifting persons receiving services for mental retardation to autism. However, shifting from other categories may affect this as well, in particular for school aged children (Eagle, 2003).
The data for ages 6-9 are sometimes discussed as being more accurate than the 3-5 data. This is logical as some diagnoses are not done until the child is older. Blaxill et al. (2003) use a median (middle number in a data set) age of diagnosis based on Kaye, del Melero-Montes, & Jick (2001) of (4.6). However, the median may not be most appropriate method for estimating how many diagnoses will be made later, as it is merely the middle number and does offer evidence as to what age, most of diagnoses are made (Jick, Beach, & Kaye, 2006) put the contemporary mean of diagnosis for Autistic Disorder at (3.1) years of age.
The 6-9 cohort numbers, have been typically higher than the 3-5 cohort, but that is not necessarily evidence for their accuracy. This could very well reflect a period of greater leniency by the DDS for whom they offer services. A review of Figure 3. supports this.
It is reasonable to predict that the level of the ages 10-13 cohort would be higher than the ages 6-9 cohort assuming that the change from ages 3-5 to 6-9 cohorts is due to delay in diagnosis. This does not seem to be the case. In fact there is typically a slight loss every three years from the 6-9 cohort to the 10-13 cohort. This attrition is unaccounted for. This may be tempting for some to assume that these children were “cured” of their autism by chelation or some other treatment. However, it would be an error to assume such an answer in the absence of better evidence. This may reflect movement out of California, it may reflect shifting to another category, it may reflect maturation of the individual or their regression towards the norm on testing and thus no longer being eligible for services under the more restrictive DDS requirements, it may simply reflect the end of a possible grace period. The DDS keeps attrition data, but does not have it organized into a database. The cost is $80.00 per hour to generate this database. To fund this is well beyond the means of this author.
In addition the prevalence rate calculated for the December quarter in 2005 equals (37) per (10,000). This is at least (10) higher, than the two epidemiology studies which came out in 2005. This could be accounted for by certain leniency from the DDS in terms of who they give services to despite the greater restrictions placed in 2003. This is an update from Croen et al. (2002) who cited the DDS prevalence rate as lower than the epidemiology.
Figure 4. shows the net changes across quarters from 1992 onwards. As per the last analysis http://interverbal.blogspot.com/2006/01/review-of-california-department-of.html
the pattern is primarily one of instability. A level change apparent in the years post 2000 still have significant variability. In fact if I was reviewing these data for a causal relation in a reversal design (baseline, introduction of independent variable, baseline) I would not conclude that the independent variable caused the change. This is doubly true, since, it is not clear where the baselines are relevant to the independent variable.
I have also included Figure 5. which is the yearly net change. This still shows instability, and includes the same problems that are discussed above. However, it also smoothed some of the instability out. This is unfortunate as instability is part of the picture of the DDS data. We must deal with this if we are to tell the whole story.
The mean of the analysis of change across quarters was (96) the standard deviation was (56). The square root of (96) = (9.7). In this case the standard deviation equals over half of mean; this indicates the high variability of the data. Ideally, the standard deviation should be near the square root of the mean.
Some of the changes may have explanations. In 1994 the DSM-IV altered the criteria for types and criteria of autism. This may account for the some of the spikes in the net change. Change of criteria is seen as a possible reason for increase in other areas of epidemiology. The changes between the World Health Organization, International Classification of Diseases (ICD) has been given as a possible reason for the increase of certain medical conditions (Friis & Sellers, 2004).
In July of 2003 a more restrictive eligibility criteria was put into place for the DDS services (Rollens, 2003). Diagnosticians may have rushed to get kids into the system ahead of the change, this may partially account for the spike in July of 2003. This is a special source of variability as is the change in the diagnostic criteria in the DSM.
A more stable source of variability comes is seen in that very few of the relative spikes happen in June or September. As has been suggested by others, this may have more to do with summer vacations than anything else. Also, the relative spikes in the data occur most frequently in March. This is not easily explained, but may simply be the time of year when the diagnosticians buckle down and do a great deal work. Other factors could influence this such as preparations for Individual Education Plans (IEP), the season for which often starts in earnest just after the Winter Holidays.
Via analysis of the data, the pattern does not seem to be truly cyclical however as is seen for monthly pneumonia and influenza mortality rates (Center for Disease Control and Prevention, 1997). Nor does these data allow us to present an epidemic threshold as we are unsure what the normal rate might be.
When we speak of autism incidence, we should speak of the youngest populations. We know that the mean age of diagnosis in the US is (3.1) years of age for Autistic Disorder (Jick et al., 2006). However, to qualify for Autistic Disorder, a child must meet criteria no later than age 3. There is no doubt that some children are diagnosed after this point. This does two things: It makes comparing data over the years difficult and it means that a 6 year old who is diagnosed with autism also met criteria for such in the past. This obscures attempts to determine case clustering.
Discussion
Threats to Validity
Since lay persons insist that these data are proof positive of certain causes for autism via “cum hoc, ergo prompter hoc” reasoning, it seems fair to subject analyses based on the DDS data for threats to internal and external validity.
There are seven threats to internal validity. The lay reports I have seen fail, to control for five of them. They do control for the other two, but those simply fail to apply in this case.
History is the first threat. It is the concept that external cultural or personal events may affect the outcome. The events of 9/11 might affect a study on depression, for example. In our case, this could be things like the study by Wakefield et al. (1998), which is shown to correlate to an autism rise in 1998 (Jick, Beach, & Kaye, 1998). This is not controlled for in the lay analyses.
Experimental Mortality, or attrition refers to the loss of a participant for various reasons. This is uncontrolled for and is evidenced in part by the discrepancy between the 6-9 cohort and the 10-13 cohort. As mentioned earlier this could be accounted for to an extent, but it has not been controlled for thus far.
Regression towards the mean, refers to the idea that over time statistical outliers tend to shift towards the mean. A child who meets criteria for the autism service category , may not do so at a later date. It is good to note that such does not imply that a child is no longer autistic, but that they simply fail to meet criteria for the DDS services.
Instrumentation, refers to the means used to measure an individual. Ideally, your instrumentation never changes over the course of a study. In our case the instrumentation did change at least twice for the data in graphs of this post. Once, in 1994, and once in 2003. This threat is uncontrolled is particularly likely to be a confound in this case.
Selection bias, refers to the including individuals in a study who bias the study in a certain direction when such do not represent the actual population. For example if I were to find some Amish persons with a low rate of autism and claim them as a representative of persons who are not vaccinated, I would be failing to control and also being guilty of using the fallacy of the Texas Sharpshooter, named after a sharpshooter who shot a cluster in a target and then drew the target around the cluster. In this case, using a voluntary service system can not possibly control for this factor, so this remains uncontrolled in the DDS analyses.
Random and Systematic error
Random and systematic errors are sources of problems that occur in epidemiology.
These are of concern in epidemiology, because they may cause us to identify or fail to identify a given pattern (Friis & Sellers, 2004). The analyses of the DDS data have failed to control for all six sources of error.
Random error involves fluctuation around a true value of a parameter. The first kind of error is Poor Precision. In this case we have a danger of the diagnosticians in the DDS assessing in different manners. This is present as there is no exact protocol aside from DSM-IV criteria and the additional more restrictive criteria implemented in 2003 for them to make eligibility judgments with.
Sampling Error is the second variety. In this case the sample is not representative of the larger population with that specific characteristic. We know that the DDS sample is not representative of the larger Autistic Disorder population in California because, of their more restrictive service eligibility criteria and due to their voluntary nature. There is the counter-intuitive problem that the prevalence via the DDS is higher than the mean prevalence post 1999.
The third kind of random error is Variability in Measurement. In this case the way we measure changes over time. This has been a concern between the ICD manuals and specific medical problems, as well the DSM manuals and autism. This is again, true as we altered the criteria to be less stringent from DSM-III to DSM-IV (Gernsbacher et al. 2005) and then tightened the criteria for legibility in the DDS system in 2003. Simply more awareness may affect the incidence of autism as evidenced by Jick et al. (2006) who found a jump in autism following the controversial Wakefield et al (1998) article and the public UK public concern over the possibility that the MMR vaccine caused autism.
Systematic errors are those problems that include a trend in the collection, analysis, interpretation, publication, or review that departs from the truth. There are three kinds; selection bias, information bias, and confounding (Friis & Sellers, 2004)
In Selection Bias, we find that the research favored a subset of a population of interest and excluded other examples in the research. The most well known example of this is the Texas Sharpshooter fallacy, which was described earlier in this post. This is present in the DDS data due to the more restrictive criteria put applied in 2003.
In Information Bias, we find that some other factors influences the accuracy of reporting. This may apply in July 2003, when the DDS autism numbers change, spiked high. This may be due to a rush by DDS diagnosticians to get as many people into the system before the more restrictive criteria were implemented. This also may account for why the DDS prevalence is higher than expected, the diagnosticians may exaggerate the severity of the developmental level of the child in order to receive services. This sub type of Information Bias is called prevarication (Friis & Sellers, 2004). This is logical as the DDS are gatekeepers for services in California. However, this is the opposite of what has been anecdotally reported by some parents of autistic children.
In the third type called Confounding, we are unable to obtain accurate results due to some other variable’s interference. Many of these are included in the five threats to internal validity that I named earlier. A Famous example is called “Simpson’s Paradox” where we receive significantly different rates between two instances of research based upon the number of persons in our samples (Rothman, 1986). This may account in part for the discrepancy between the high variability seen in recent years in the incidence calculated via the DDS data and what is seen for incidence via the epidemiology which has been stable in the recent years (Jick et al., 2006).
Conclusion
Future analyzers of the DDS data should be aware that these data are not recommended for this purpose according to the DDS. The cohort of primary interest should be the 3-5 age group. The data do not resemble the existing epidemiology and therefore do not seem externally valid. They do not evidence a pattern that clearly discernable and are quite variable between quarters. They can not support or refute an epidemic threshold level. They can not give an accurate case cluster analysis. They fail to account for five out of seven significant sources of internal validity. Finally, due to their observational nature, they not establish causation.
To conclude, Rollens (2006) poses a question that was answered in a previous post on this blog. Now I would like to take the chance to reciprocate and ask a question: What is the ethical standing of using the California DDS data when the DDS has stated that they should not be used for this purpose and when they do not resemble other existing epidemiology?
Notes: I want to thank the US Census Bureau and the California DDS for very generously fielding my questions.
References
American Psychiatric Association. (1980). Diagnostic and Statistical Manual ofMental Disorders, Third Edition. Washington, DC: American Psychiatric Association; 1980.
American Psychiatric Association. (1987). Diagnostic and Statistical
Manual ofMental Disorders, Third Edition, Revised. Washington, DC: American Psychiatric Association; 1980.
Manual ofMental Disorders, Third Edition, Revised. Washington, DC: American Psychiatric Association; 1980.
American Psychiatric Association. (1994). Diagnostic and Statistical
Manual ofMental Disorders, Fourth Edition. Washington, DC:
American Psychiatric Association; 1994.
Manual ofMental Disorders, Fourth Edition. Washington, DC:
American Psychiatric Association; 1994.
American Psychiatric Association. (2000). Diagnostic and Statistical Manual ofMental Disorders, Fourth Edition, Text Revision. Washington, DC:
American Psychiatric Association; 1994.
Autism: The Hidden Epidemic. MSNBC. March 3, 2005.http://www.msnbc.msn.com/id/6844737/.Accessed September 16, 2005.
Angel, M. (1990). The interpretation of epidemiological studies. Editorial, New England Journal of Medicine. 332, 823-825.
Baird, G., Charman, T., Baron-Cohen, S., et al. (2000). A screening instrument for autism at 18 months of age: a 6 year follow-up study. Journal of American
Academy of Child and Adolescent Psychiatry 39, 694-702.
Academy of Child and Adolescent Psychiatry 39, 694-702.
Bertrand, J., Mars, A., Boyle, C., Bove, F., Yeargin-Allsop, M., & Decoufle, P.
(2001). Pediatrics, 108, 1155-161.
(2001). Pediatrics, 108, 1155-161.
C. H. (2006). Department of Disability Services. Personal Communication.
Chakrabarti, S., & Fombonne, E. (2001). Pervasive developmental disorders in preschool children. Journal of the American Medical Association, 285,
3093-3099.
3093-3099.
Chakrabarti, S., Fombonne, E., (2005). Pervasive developmental disorders in preschool children: confirmation of high prevalence. American Journal of
Psychiatry, 162(6), 1133-1141.
Psychiatry, 162(6), 1133-1141.
Centers for Disease Control and Prevention. (1997). Weekly Influenza and Pnuemonia Mortality. MMWR.
46, 327.
46, 327.
Department of Developmental Services (2006). Quarterly Client Characteristics Reports.http://www.dds.ca.gov/FactsStats/quarterly.cfmAccessed Friday January 13, 2005.
Department of Developmental Services (2005). Data Interpretation Considerations and Limitations.http://www.dds.ca.gov/FactsStats/pdf/CDER_QtrlyReport_
Consideration_ Limitations.pdfAccessed Friday January 13, 2005.
Consideration_ Limitations.pdfAccessed Friday January 13, 2005.
Fombonne, E. (2001). What is the prevalence of Asperger syndrome? Journal of Autism & Developmental Disorders, 31: 3, 363-364.
Fombonne, E. (2001). Is there an epidemic of autism? Pediatrics.Vol 107 (2), 411-412.
Fombonne, E., Simmons, H., Ford, T., Meltzer, H., Goodman, R. (2001). Prevalence of pervasive developmental disorders in the British national survey of child mental health. Journal of the American Academy of Child & Adolescent Psychiatry 40, 820-827.
Fombonne, E. (2002). Prevalence of childhood disintegrative disorder (CDD). Autism 6, 2, 147-155.
Fombonne, E. (2003). Epidemiological surveys of autism and other pervasive developmental disorders: an update. Journal of Autism and Developmental Disorders. 33, 365-382.
Friis, R. H., Seller, T. A. (2004). Epidemiology for public health practice, 3rd ed. Sundbury, MA: Jones and Bartlett Publishers.
Gernsbacher MA, Dawson M, & Goldsmith HH. (2005).Three reasons not to believe in an autism epidemic. Current directions in psychological science, 14 (2), 55-58.
Honda Shimizu, Y., Rutter, M. (2005). No effect of MMR withdrawal on the incidence of autism: a total population study. Journal of Child Psychology and Psychiatry, vol 46 (6), 572-579.
Individuals with Disabilities Education Act. (1990). Public Law 101-476, U.S.C.
Jick H, Beach KJ, Kaye JA. Incidence of autism over time.Epidemiology. (2006). Epidemiology, 17(1), 120-121.
Kaye, J. A., del Melero-Montes, M., & Jick, H. (2001). Mumps, measles, and rubella vaccine and the incidence of autism recorded by general practitioners: A time trend analysis. BritishMedical Journal, 322, 460–463.
Kielinen, M., Linna, S. L., & Moilanen, I. (2000). Autism in Northern Finland. European Child and Adolescent Psychiatry, 9, 162-167.Laidler, J. (2005). US Department of Education data on "autism" are not reliable for tracking autism prevalence. Pediatrics, 116 (1), 120-124.
Last, J. M. (1995). A dictionary of epidemiology, 3rd ed. New York, NY: Oxford University Press.
Magnusson, P., & Saemundsen, E. (2001). Prevalence of autism in Iceland. Journal of Autism & Developmental Disorders 31: 153-163.
Mandall, D. S., Novak, M. M., Zubritsky, C. D. (2005). Factors associated with age of diagnosis among children with autism spectrum disorders. Pediatrics,Vol 116 (6), 1480-6.
Powell, J., Edwards, A., Edwards, M., et al. (2000). DevelopmentalMedicine and Child Neurology, 42, 624–628.Rollens, R. (2006) California Reports: New Autism Cases at 4 Year Low. Schafer Autism Report, 10 (7). Thursday, January 12, 2006, http://www.sarnet.org/Accessed Accessed Friday January 13, 2005.
Rollens, R. (2004). California Autism Intakes Down Slightly -- Factors Discussed. Schafer Autism Report, 8, 165. Wednesday, October 20, 2004, http://www.sarnet.org/Accessed Accessed Friday February 4, 2005.
Taubes, G. (1995). Epidemiology faces its limits. Science, 269, 164-9.
Taylor B, Miller E, Farrington CP, et al. Autism and measles, mumps, and rubella vaccine; no epidemiological evidence for a causal association. Lancet, 353, 2026-2029.
United States Census Bureau. (2006). Personal Communication.
Wakefield, A., Murch, S., Anthony, A., Linnell, J., Casson, D., Malik, M., Berelowitz, M., Dhillon, A., Thomson, M., Harvey, P., Valentine, A., Davies, S., & Walker-Smith, J. (1998). Heallymphoid-nodular hyperplasia, non-specific colitis, and pervasive developmental disorder in children. Lancet, 351, 637-641.
Winklestein, W. (1995). Invited commentary on “Judgement and Causal Inference: criteria in epidemiological studies”. American Journal of Epidemiology, 141, 699-700.
World Health Organization. (1992). International Classification of Diseases, 10th Revision (ICD-10). Geneva, Switzerland: World Health Organization; 1992.
Yeargin-Allsopp, M., Rice, C., Karapurka, T., Doernberg, N., Boyle, C., Murphy, C. (2003). Prevalence of autism in a US metropolitan area. Journal of the American Medical Association, 289, 49-89.
5 Comments:
Most excellent, Interverbal. Enormous thanks to you for the time you spent and your detailed analysis. I am impressed by the style of your writing, in that not only are you presenting your argument (with cites, etc.), but also you are reminding many of what contitutes statistical methods and analyses. I found myself, in reading your blog post, saying several times to myself: "Oh, yes, of course, I'd forgotten that rule."
So, in short it is brilliant. Thank-you also for the final question you present in re: the ethics of using data in which the collector/keeper of the data explicitly states should not be [mis]used for certain purposes.
HJ
Thank you, Interverbal.
Autism Diva thinks that there's something like "regression toward the mean" or rather progression toward the normal over time with autism, so that if you could take children born in say, 1920 and 1930, and test them, a large number would come up as autistic, but left to their own devices and with whatever therapies (and/or tortures) were provided (imposed on) them, including normal parenting... many would get to the point that they wouldn't need special services.
They might not be living a super "normal" life, but like an uncle by marriage of Autism Diva's, they might have a job and a little house or apartment and not really get any services. That uncle never married and ended up being taken advantage of terribly by a man he worked with, now he lives with a sister of his.
Some of the eventual functionality of autistics depends on how much they feel loved and valued when they are little.
Then there's the whole thing of how many of those cohorts from the 1920's and 30's died. Some of the higher functioning spectrum guys probably died, like Bubba Blue (the character) in Forrest Gump in wars, and some killed themselves, and some died of neglect and of being drugged in state hospitals and choking on their own vomit, plus just the fact that the drugs are heinously dangerous in other ways.
Autism Diva has given the experience before of a man who is about 40 years old today who was diagnosed as having "childhood schizophrenia" in the early 1970's, when he was 4 or 5 years old. He was autistic, not schizophrenic. Today he is married, has two girls and a business he owns. He's Autism Diva's friend's brother. He's a very irritating, socially inept person, but he can drive a car and run a business and is pretty successful. That man is the brother of Autism Diva's friend who has an autistic son dxd at age 12 and added to the California DDS load about a year ago. The little brother of that boy is 2+ now and is a sweet little boy with a speech delay, but is not autistic.
Edit:
"so that if you could [go back in time and] take children born in say, 1920 and 1930, and test them,"
... like time travel... not possible... it's a thought experiment. :-)
Excellent!
Hi Citizen Cain,
I am no stranger to Citizen Cain's blog. I thought your work on this stuff was rock solid.
Post a Comment
<< Home